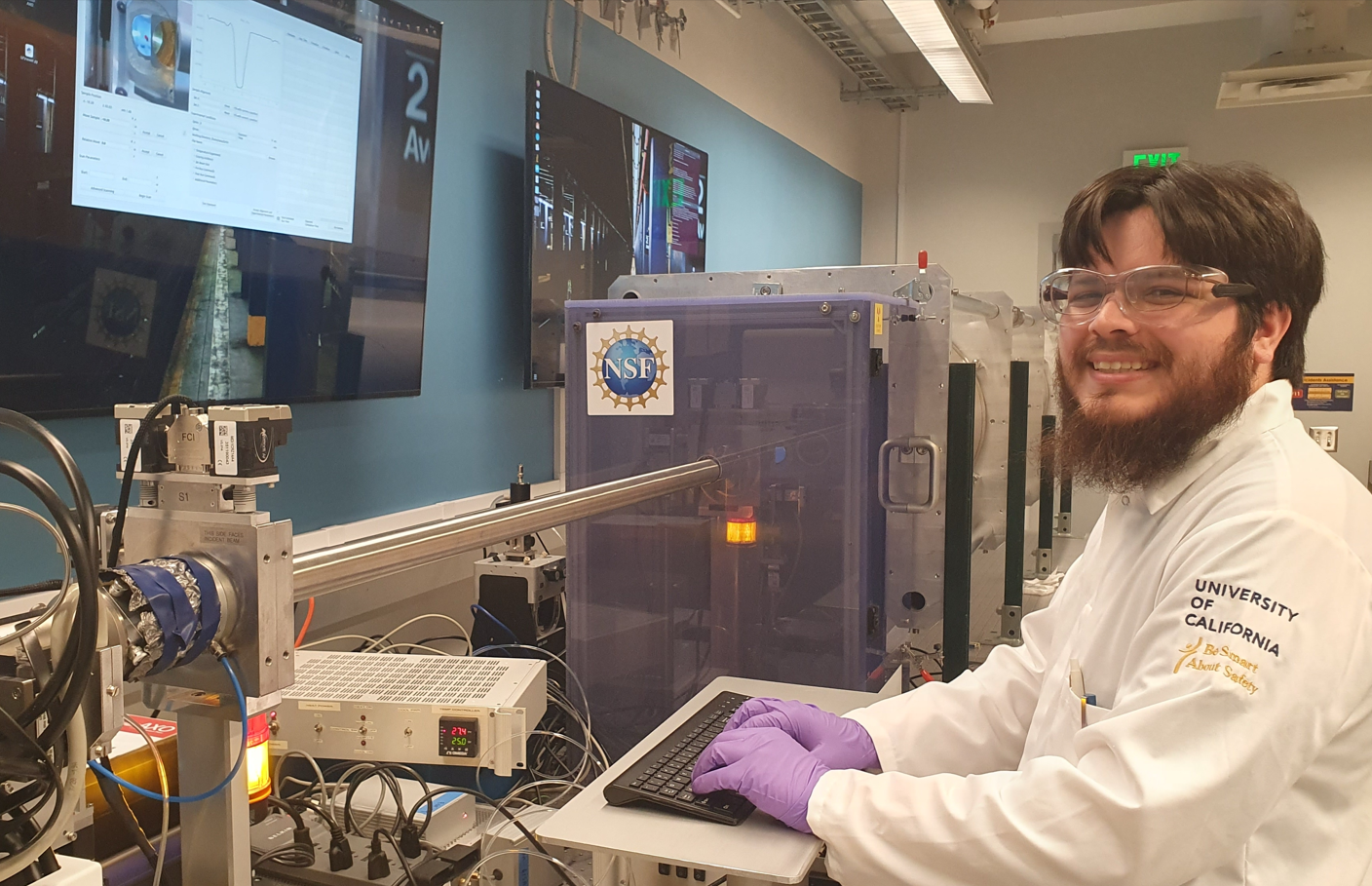
Small Angle X-ray Scattering, or SAXS, is a powerful tool for characterization of materials on the nanoscale. SAXS experiments are often hindered by long exposure times due to low source flux and/or scattering contrast of the system. Advances in technology have increased the fluxes of in-house X-ray systems substantially over the last couple decades, however, high-end X-ray diffractometers are expensive and contain a brilliance several orders of magnitude lower than synchrotron radiation, which is often insufficient for most experiments. Deep learning may be able to resolve low-intensity experiments through multi-step forecasting. We constructed a training set using a library of time monitored experiments run through the BioPacific SAXS machine. By training a multilayer perceptron (MLP) on this training set, the MLP would then predict what the sample would look like at a given flux. However, the MLP is continuing to make inaccurate predictions, due to various potential reasons such as the weight rigidity. We have attempted to adjust the MLP to account for the varying scattering profiles in the data. One such alternative is to use a different model, such as the Bayesian Neural Network, which uses a probability distribution for weights that may account for these various scattering profiles.