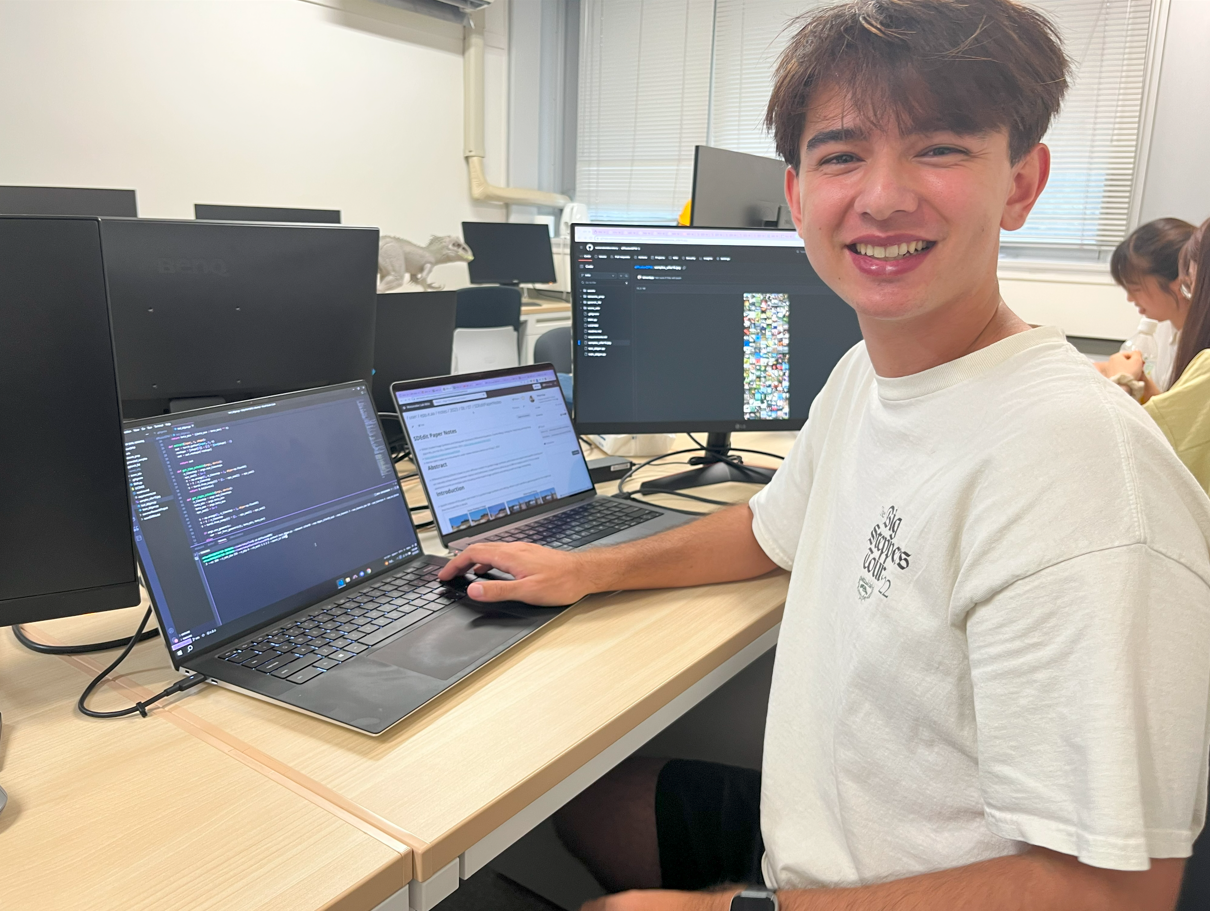
Dynamic Projection Mapping (DPM) is a captivating visual technique that involves rendering
and projecting content in real time which aligns with the shape and movements of physical
objects in the real world, creating an immersive and interactive visual experience. In order to
achieve sufficient alignment between projection and object, projections need to be rendered
within 1-2 milliseconds. In the field of Artificial Intelligence, Diffusion Probabilistic Models
(Diffusion Models) provide state of the art performance, particularly in high quality image
generation. The one major downside of these models is their slow generation time, with models
often taking dozens of seconds to generate a single image. Our research focuses on bringing
together these two cutting edge fields by bridging the time gap between the high speed
requirements of DPM and slow generation times of Diffusion models. Since this gap is very
large, initial steps towards the goal are achieved by testing with static high speed projection in
order to confirm the hypothesis that the approaches from traditional DPM techniques can apply
to these Diffusion Models. Our acceleration strategy utilizes nuances of human visual perception
with high-frame-rate displays. We've developed a method that enables viewers to perceive
images from these Diffusion Models as higher fidelity, even when generated rapidly and at a
lesser quality. Our results confirmed the hypothesis that the methods proposed can successfully
generate an image that, at high frame rates, can be perceived as a higher quality image. The
proposed method obtains acceleration proportional to the number of timesteps reduced in the
Diffusion Model and the acceleration is done in such a way that is applicable to the high speed
projection techniques of DPM. This work lays the groundwork for future research in bringing
diffusion models to DPM by showing the capabilities of the proposed acceleration techniques in
accelerating the models.