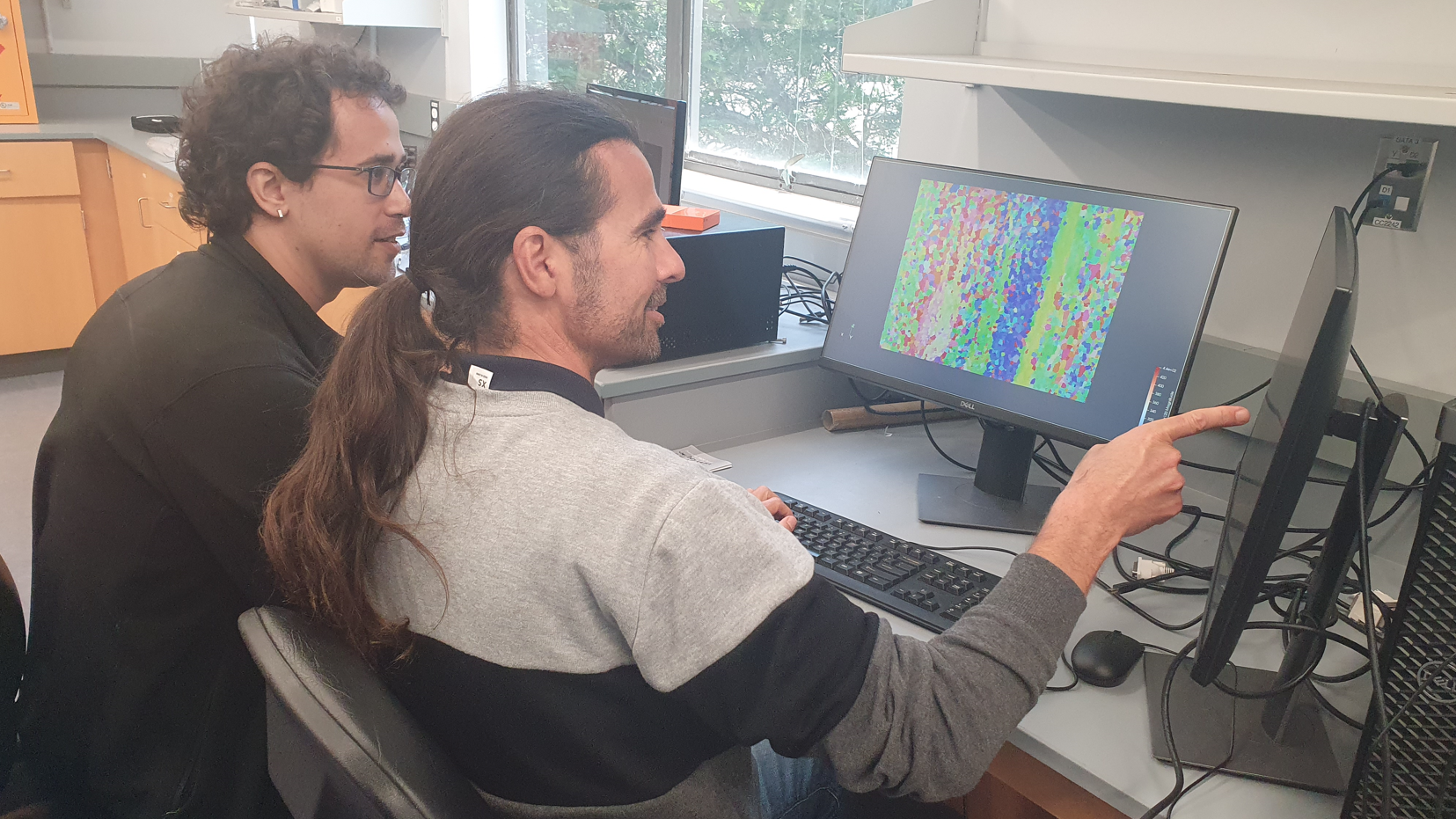
Studying the relationship between the microstructure of a polycrystalline material and its properties requires the identification of individual grains within the structure. Knowledge of this relationship can be used in materials research, such as the development of grain level finite element analysis simulations that help to understand why some materials yield prematurely under certain loading conditions. Currently, for Electron Backscatter Diffraction (EBSD) maps, this is a manual process that can take weeks to months to complete. Our research aims to reduce this process to less than an hour using computer vision and machine learning (ML). A combination of adaptive thresholding and contour mapping of 49 inverse pole figure (IPF) maps identified more than 80% of grains with an intersection over union (IoU) of greater than 80% using the Open-Source Computer Vision Library (OpenCV). IoU represents the percentage overlap between the model’s segmentation and the manual segmentation performed by a human (i.e., the ground truth). After pre-processing, the data can be used as input to Meta’s Segment Anything Model (SAM). This ML model has been pre-trained on 11 million images and 1.1 billion segmentation masks, and provides zero-shot generalization to novel segmentation tasks. Once an average IoU above 98% is achieved the 2D segmented data can then be used to identify 3D grains within the sample.